Revolutionizing Business with Control System Machine Learning
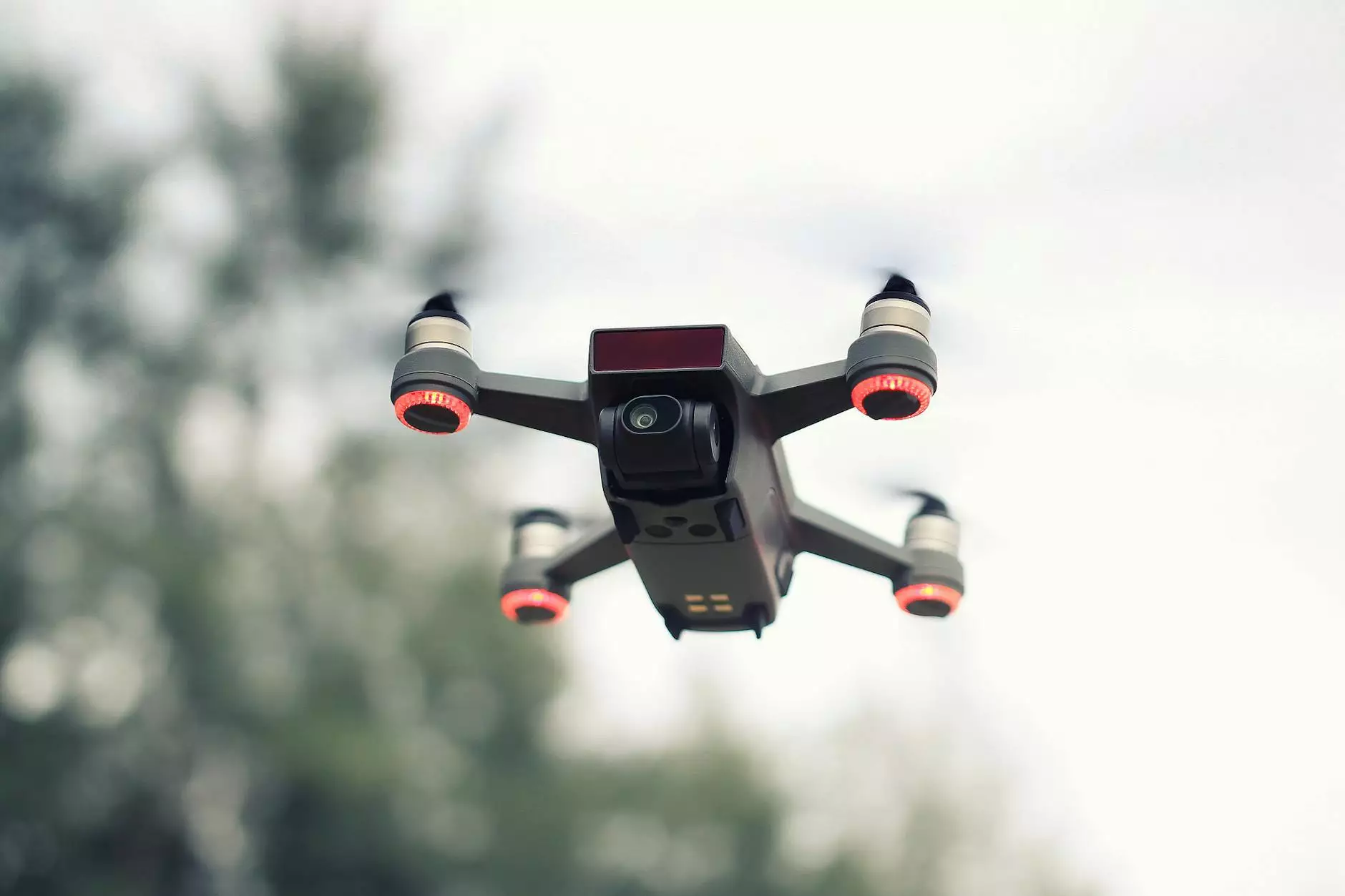
In an era where technology governs the heartbeat of every successful enterprise, control system machine learning stands as a transformative force. Businesses today are navigating an increasingly complex landscape characterized by rapid technological advancements and evolving consumer demands. The ability to effectively utilize machine learning within control systems not only enhances operational efficiency but also fosters innovative approaches in content management services, business process automation services, and data governance systems.
Understanding Control System Machine Learning
At its core, control system machine learning refers to the integration of machine learning algorithms with control systems designed to manage dynamic processes. This includes the automation of data analysis, predictive modeling, and adaptive control mechanisms. The objective is to improve decision-making processes, optimize system performance, and ultimately drive business success.
What Makes Control Systems Important?
- Efficiency: Streamlined operations lead to significant reductions in costs.
- Scalability: As businesses grow, control systems equipped with machine learning adapt to increased data volumes and complexities.
- Precision: Enhanced data analytics leads to better forecasting and decision-making.
- Adaptability: Machine learning algorithms can continuously learn from new data, ensuring that control systems remain effective even as conditions change.
The Role of Machine Learning in Business Process Automation
In the context of business process automation services, machine learning algorithms can analyze vast datasets, identify patterns, and make predictions that aid in automating repetitive tasks and decision-making processes. This leads to:
Key Benefits of Automation through Machine Learning
- Time Savings: Automating mundane tasks allows employees to focus on high-value activities that require critical thinking and creativity.
- Cost Efficiency: Reducing labor costs while improving service delivery translates into significant savings.
- Error Reduction: Machines are less likely to make errors compared to humans in repetitive tasks, ensuring consistency in outputs.
- Improved Customer Experience: Faster processes lead to enhanced customer satisfaction through timely service delivery.
Enhancing Data Governance with Control System Machine Learning
Control system machine learning significantly impacts data governance systems by enabling better data quality management, compliance, and security measures. As businesses handle increasing amounts of data, the need for robust governance frameworks is paramount. Machine learning enhances governance in a variety of ways:
How Machine Learning Fortifies Data Governance
- Data Quality Control: Machine learning can identify and rectify inconsistencies in datasets automatically.
- Risk Management: Predictive analytics can foresee potential compliance issues before they occur, allowing businesses to act proactively.
- Access Management: Intelligent systems can learn access patterns and identify anomalies, thus enhancing data security.
- Continuous Improvement: Machine learning models can evolve over time, ensuring that data governance practices remain relevant and effective.
Content Management Services Enhanced by Machine Learning
Content management services, pivotal for modern businesses, are also enhanced through the application of control system machine learning. By utilizing these advanced technologies, businesses can streamline content creation, management, and distribution processes. Here’s how:
Machine Learning in Content Management
Machine learning facilitates better content management in the following ways:
- Automated Content Tagging: Algorithms can automatically tag and categorize content based on inherent attributes, improving searchability.
- Personalization: Analyzing user behavior allows businesses to tailor content to individual preferences, enhancing user engagement.
- Content Strategy Development: Data-driven insights help marketers understand what types of content resonate most with their target audience.
- Performance Analysis: Automated systems can evaluate content performance continuously, providing insights that drive future strategies.
Integrating Control System Machine Learning into Business Strategies
The integration of control system machine learning into broader business strategies can create a competitive advantage. Here are some steps businesses can take to harness the power of this technology:
Steps to Integration
- Assessment of Current Systems: Analyze existing control systems to identify gaps where machine learning can be applied.
- Data Collection: Ensure that valuable data is being collected correctly and comprehensively.
- Algorithm Development: Collaborate with data scientists to develop machine learning models tailored to specific business needs.
- Testing and Validation: Rigorously test machine learning applications to ensure effectiveness and reliability.
- Deployment and Monitoring: Post-deployment, monitor the systems continuously to optimize performance and adapt to changes.
Real-World Applications of Control System Machine Learning
Numerous industries are leveraging control system machine learning to innovate and enhance their operations. Here are a few examples:
Industry Examples
- Manufacturing: Predictive maintenance systems detect anomalies in machinery, allowing for proactive maintenance and reducing downtime.
- Finance: Machine learning algorithms analyze market trends to provide insights for trading strategies and risk assessments.
- Healthcare: Patient monitoring systems use machine learning to analyze vital signs and forecast potential health issues before they arise.
- Retail: Retailers use machine learning for inventory management, helping to predict product demand and optimize stock levels.
Challenges and Considerations in Implementing Machine Learning
While the benefits of implementing control system machine learning are manifold, businesses must also navigate several challenges. Awareness and proactive strategies can mitigate these challenges:
Common Challenges
- Data Privacy: Ensuring that customer data is handled ethically and in compliance with regulations is paramount.
- Model Bias: Machine learning models can develop biases based on the data they are trained on, leading to inaccurate outcomes.
- Integration Complexity: Merging new machine learning systems with existing infrastructure can be technically challenging.
- Skill Gap: A lack of skilled professionals in data science and machine learning can hinder implementation.
The Future of Control System Machine Learning in Business
The future of control system machine learning in business looks promising. As technology evolves, we anticipate several trends that will shape this landscape:
Emerging Trends
- Increased Automation: Businesses will continue to automate processes, driven by efficiency demands.
- AI and Machine Learning Fusion: The combination of AI and machine learning will lead to even smarter systems.
- Personalized Experiences: Companies will focus on using data to create highly personalized customer experiences.
- Ethical AI Implementation: Transparent and ethical approaches to AI will gain prominence, reflecting consumer preferences and societal values.
Final Thoughts
In conclusion, the deployment of control system machine learning is not just a technological upgrade; it is a strategic imperative for businesses aiming to thrive in a competitive environment. By improving processes, enhancing data governance, and revolutionizing content management, machine learning equips organizations to respond adeptly to market demands and operational challenges. As companies continue to harness the potential of machine learning, they will not only improve their service delivery but also lay the groundwork for continuous innovation and growth. Embracing this technology is crucial for any business seeking to secure its position in the future marketplace.